Quantum Computing Undermined by Radical Uncertainty: Part One
Standard Chartered is apparently set to use ‘quantum machine learning’ to pursue problems of uncertainty in finance and investing, and software company Phasecraft will use quantum approaches in pharmaceuticals research.Standard Chartered will probably fail. Phasecraft’s focus on pharmaceutical’s has a better chance of success.This is because the two projects deal with completely different types of uncertainty.
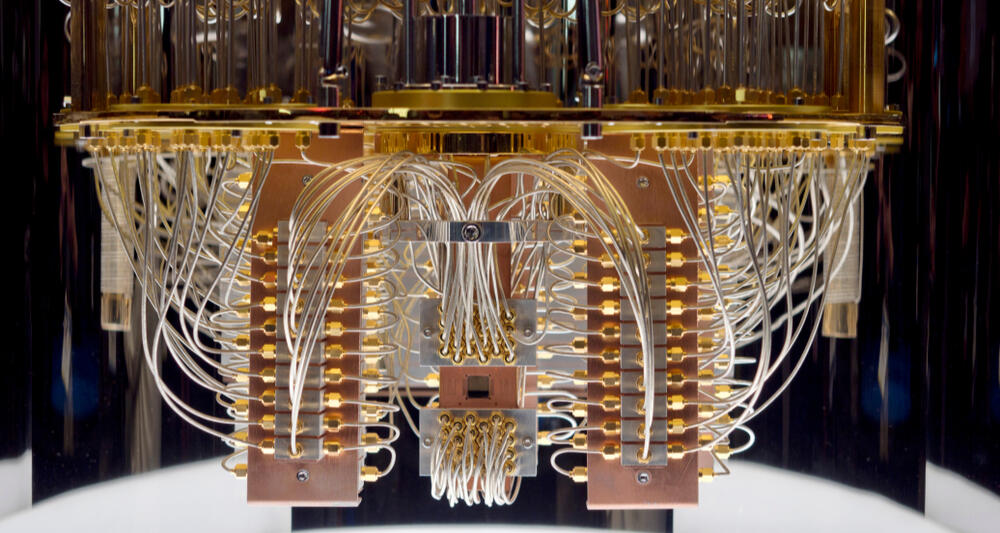
This seemingly innocuous division is actually a chasm, and one which quantum computing power does not bridge.So what are these different types of uncertainty, and why can quantum machine learning help overcome one but not another? Also, is there anything we can learn from what humans already do with their own 'biocomputers' (their brains) to help cope with the type of uncertainty this technology is not so useful for?
Roulette and missiles: different problems
Clear your mind for a moment. Now imagine you are present in the following two scenarios, each from a similar historical period - the 1960’s - and the same country - America.The first is standing at a roulette table next to, say, Frank Sinatra, in Las Vegas, weighing the potential rewards of betting your life savings on the number 35. The second is a strategic meeting in the Cabinet Room of Jack Kennedy’s EXCOMM advisers at the height of the Cuban Missile Crisis.At the roulette table, you are thinking about the likelihood of winning or losing a lot of money. You also feel extremely uncertain. If you’re able to remain calm, you might be considering probability distributions and expected returns calculations - trying to determine a likelihood based on the rules of the game. And perhaps worrying about what to say to your famous but shady companion.
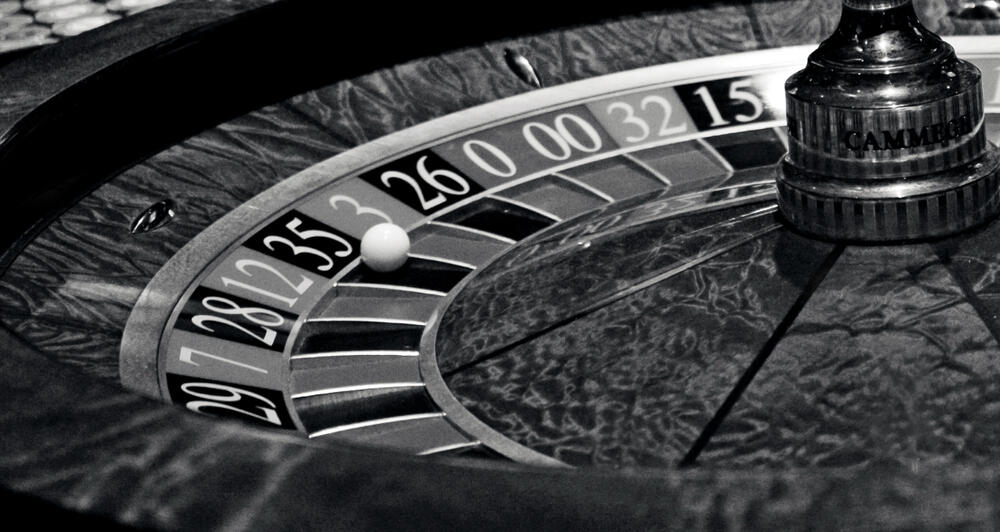
The roulette wheel we are stood at with Mr Sinatra.
At the EXCOMM meeting, you are witnessing experienced politicians, military advisers, diplomats and intelligence officials deliberate. They are trying to decide whether or not to launch air raids on identified nuclear missile sites in Cuba, which are preparing for a potential launch on the US mainland. The clock is ticking.
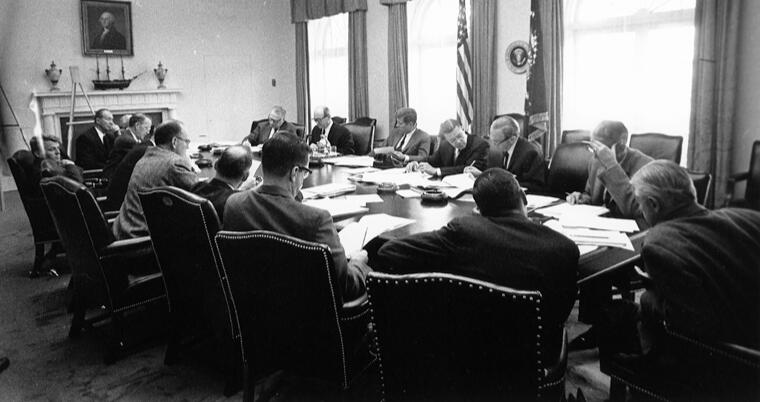
EXCOMM meeting at the White House Cabinet Room during the Cuban Missile Crisis in October 1962.
These two scenarios are both dealing with uncertainty, and they are both trying to manage it:
For the roulette table: “What is the likelihood that, if I bet my savings on 35, I will be able to buy a new house, or I will be homeless?”
For the EXCOMM meeting: “Will bombing offensive missile sites in Cuba eliminate the threat of a nuclear attack from that island, or will this move be interpreted by Moscow as a dramatic escalation and result in a Soviet move against Berlin, and ultimately a full nuclear exchange?”
But they are also dealing with very different types of problem.
The first, the game of roulette, is addressing a stationary problem: the rules are set and they won't change. No matter how many times you play the game, the chances of winning or losing will be the same, and that means we can actually assign numerical values to those chances.In fact, to cope with this problem today, we could run it through a simple computer and it would tell us the probable outcomes of lots of different bets on the roulette wheel. We could even plot those results as neat, intuitive distributions on a graph. We could then bet accordingly (provided our computer wasn’t spotted).The second, the EXCOMM meeting, is dealing with a non-stationary problem. The rules are not set, they are unpredictable and are changing with every move that is made. This means that offering President Kennedy probability distributions for the potential outcomes of the decisions he’s considering would be at best useless and, at worst, misleading. This is because every single element of this problem, from how ready the Cuban missiles actually are, to what the Soviets are thinking, could change. Further, there could be things we are simply not aware of that are at play.To cope with this problem, the President has listened to his advisers intently, drawn on his assimilated knowledge and hard won experiences like the Bay of Pigs debacle a year before, and thought about how Premier Kruschev might be thinking. In October 1962 he got it right, just.These problems are, firstly, contrived and, secondly, extreme. But they demonstrate some key differences between types of uncertainty. Also, they reveal why one type of uncertainty - that created by non-stationary problems, cannot be addressed adequately by computers and machine learning tools.
Ramifications and ‘hype’ intersections
Problems arise because people conflate these two types of uncertainty and impose stationary parameters on non-stationary problems and disciplines. What’s more confusing is that modern computing power is now being presented as the solution to uncertainty caused by non-stationary problems: specifically, the separate ‘hypes’ around quantum computing and AI machine learning by neural nets appear to have intersected, and this could have damaging consequences.
This article is the first half of Rebbelith's exploration into how quantum computing is undermined by Radical Uncertainty.It will explore the viability of quantum machine learning as a tool for coping with this uncertainty by focussing on two key points:
1. Why finance and investing will not benefit from quantum machine learning as expected and how, because of non-stationarity, its application may embed and magnify risks.2. Why some uncertainties, like those faced in pharmaceuticals development, make a viable application for quantum machine learning.
Read on below
Ignoring Keynes and dressing up non-stationary problems as stationary puzzles: why Standard Chartered will fail in using quantum machine learning
The key uncertainties in finance and investing aren't stationary problems, they are unique events. They are not subject to set rules and variables: we can't make completely accurate predictions about things like interest rates, asset prices or the economy.What happened in the past does not mean the same will occur in the future. Furthermore, models that try to deliver some certainty in finance and economics must hold numerous variables constant (basically remove their effects) in order to do so. Consider the Capital Asset Pricing Model or the Markowitz Portfolio Model as some well known examples.Even though many in the industry would argue that they can make predictions or cope with ‘risk’ in finance, they can't: there are too many variables and the rules are not as set as people say they are (refer someone who disputes this to banks’ risk models in 2008). The problems are distinctly non-stationary, and some people make money whilst others lose it. Furthermore, those that lose it are often possessed of powerful computers at genuinely clever hedge funds.So it's important to appreciate why these powerful computers, and even quantum computing power, cannot cope with these problems.
Standard Chartered’s Quantum Born Machine
AI neural net machine learning processes ‘learn’ by observing patterns in data. To do this they need to be fed immense datasets, covering all possible conformations, to train on in order to pick out patterns reliably. But finance faces a significant problem in that available datasets have too few real-world sample data with which to do this, and those data are based on the past.Alexei Kondratyev, managing director at Standard Chartered, was reported as saying one of their first tasks for the quantum computer will be creating a “Quantum Circuit Born Machine” to create ‘synthetic’ data.The aim of a Born machine is ‘generative modelling’, which takes a dataset with few points (like those currently available in finance), tries to extract the underlying probability distribution, and then creates more datapoints within the inferred distribution. These are then used to provide input for an AI neural net machine learning process. Essentially, Born machines allow you to generate new or ‘synthetic’ datasets by interpolating between real data points, and these datasets can be used to train AI neural net machine learning tools.
AI machine learning + quantum computing VS John Maynard Keynes
John Maynard Keynes introduced the concept of ‘Radical Uncertainty’ into predicting the future, emphasising that probability distributions could not be assigned to future events in non-stationary systems: as we saw earlier, when we were observing the EXCOMM meeting during the Cuban Missile Crisis, handing President Kennedy probability distributions assigned to possible choices would have been misleading - so much so that it may have resulted in nuclear war. His team succeeded without them. Why?In essence what we saw at that meeting was that systems should be designed to cope with uncertainty, rather than producing neat but false probabilities.The Born machine is powerful when applied to a reproducible or stationary physical system, which is what it is designed for (we will explore its applications in pharmaceuticals development shortly). But it is not good with historical economic and financial datasets. These are neither reproducible, nor stationary, and probably do not have stable underlying probability distributions.Standard Chartered’s Born machine will be creating synthetic datasets that may, ultimately, be worthless. This is because they will be based on original samples from historical, non-stationary, financial and economic data. Simply, the perennial problem that the future may not resemble the past will not be overcome, and this will blur the distinction between ‘real’ data points and those generated by the Born machine.There are, however, problems that computers can have a significant impact upon. They are by no means simple.
Fiendishly complex but stationary problems - why pharmaceuticals development can benefit from quantum machine learning
Many stationary problems are incredibly complex and their solutions have eluded extremely bright people, sometimes for decades. But their nature means modern computing power can be put to work productively. An example of such a decades-long challenge is the ‘protein folding problem’ and its role in curing diseases.
Making shapes, spontaneously
Almost everything the body does is dependent on proteins and how they change. The capability of a particular protein depends on its 3D shape, like the Y-shaped proteins used by our immune systems that ‘hook’ onto viruses and bacteria. The blueprint for such proteins is contained in our DNA, errors in which can make proteins form incorrectly, and cause diseases.Proteins ‘fold’ into their complex 3D shapes spontaneously and in milliseconds, and scientists have wrestled with predicting these shapes for decades in what’s known as as the ‘protein folding problem’.
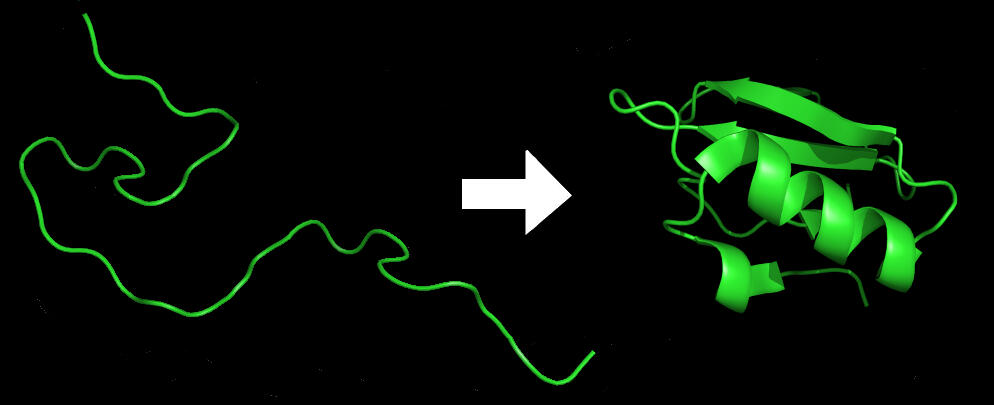
Illustration of the process of protein folding.
The ‘folding problem’ is important because if a protein’s 3D structure can be known, its job in a cell can be predicted. That means drug developers can create solutions that collaborate with a protein’s specific shape.
Putting Born machines and AI neural networks to work
Scientists have spent five decades using trial and error experiments to determine the shape of proteins, but this has taken too long and cost hundreds of thousands of dollars to determine even the structure of single proteins.But the cost of genetic sequencing has fallen rapidly. This has created an abundance of real-world data that can be used to feed Born machines, and subsequently AI neural networks. Essentially, this permits scientists to predict the structure of a protein computationally just from its genetic code.The DeepMind team have already trained neural networks to deal with this problem, and the system they built yielded very accurate 3D protein shapes. Their work has the potential to assist researchers of rare diseases and contribute to efficient drug discovery, improving the lives of millions of people around the world.
So, what have we found?
Essentially, we've seen that there are types of uncertainty which powerful computers cannot cope with, that these problems are sometimes crucially important and that finance is one of them: we have seen the challenges posed by non-stationarity.We have seen that whilst Standard Chartered will struggle to apply quantum machine learning to their non-stationary problems, Phasecraft is probably on the money with pharmaceuticals research.We've found that just because a problem is stationary - like protein folding- it is by no means simple. But it's in these problems that AI neural nets fed by Born machines can probably have the most impact.
In Part Two...
The second half of Rebbelith's exploration into uncertainty will discuss what, if we are to make better decisions and retain a competitive edge, we must consider.We will show that the ability to cope with real-world problems will not be found in an unobservable AI neural net process, however large a synthetic, biased dataset is used to train it on.In this second part, readers will see that systems designed to help us cope with uncertainty in non-stationary problems, like business, must accommodate the way the world actually is, and the way humans actually think.
About Rebbelith
Rebbelith developed technology to integrate every aspect of campaign and communications strategy into a single service. Our solution uses interactive visual models to help strategists influence complex discussions in the media and map their target stakeholders with new precision.These models are dynamic to accommodate a changing world and help experienced teams navigate uncertainty, giving them a truly competitive edge: not only can they raise awareness of their goals sustainably, but they can now also enter larger media debates more productively, and actually change their structure.Rebbelith achieves this by analysing global news at speed, at scale and internationally. Our systems then explore that media activity to reveal the networks and narratives connecting stories and stakeholders. Finally, these data are converted to condensed visual intelligence, allowing a single strategist to assimilate months of media discussion in minutes, without the need for large research teams.Rebbelith can operate anywhere in the world.
Contact Us
Read Part Two
Rebbelith Research
© 2021 Rebbelith Ltd. All rights reserved. UK registered company 12447815